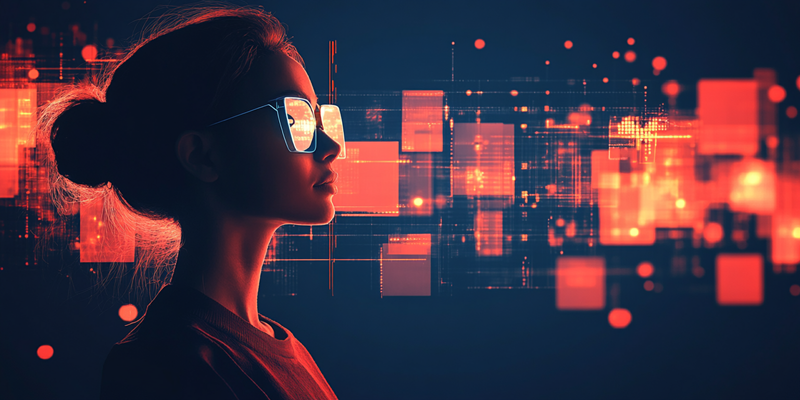
Marketing has always relied on data to make informed decisions, but the advent of advanced analytics has redefined how data can be leveraged to drive impactful outcomes. While traditional marketing analytics methods have served their purpose for decades, advanced analytics introduces a new paradigm—offering deeper insights, better personalization, and enhanced efficiency.
This article explores the types of marketing analytics needed to support a modern advanced analytics-enhanced marketing program, contrasting these approaches with traditional techniques and highlighting the incremental benefits of advanced analytics driven solutions.
Traditional Marketing Analytics vs. Advanced Analytics
Traditional marketing analytics often relied on historical data and qualitative or minimally quantitative approaches. These methods were often sufficient for broad insights but fell short of providing granular, actionable recommendations.
For example, traditional heuristic-driven segmentation methods often grouped customers into broad qualitatively derived and often ill-conceived categories. Similarly, most traditional campaign response analytics contain minimal quantitatively grounded insights connecting past behavior and future actions.
These approaches all lie relatively low on the Analytics Maturity curve; in the “Descriptive Analytics” region where the basic reporting on “what happened?” or “what’s happening?” takes place. Our full discussion of the Analytics Maturity curve can be found here.

Advanced Analytics, in contrast, introduces AI and machine learning (ML) tools capable of investigating vast datasets, identifying nuanced patterns, and adapting to new information in real time. Moreover, these new advanced analytics based approaches provide insights that extend well beyond the capabilities of traditional methods, and are capable of delivering Diagnostic, Predictive, and Prescriptive insights that enable organizations to optimize their decision making towards desired outcomes.
Let’s dive into the different types of analytics that underpin traditional marketing programs, and discuss the way that new advanced analytics enhanced tools can provide enhanced utility.
- Market Basket Analysis
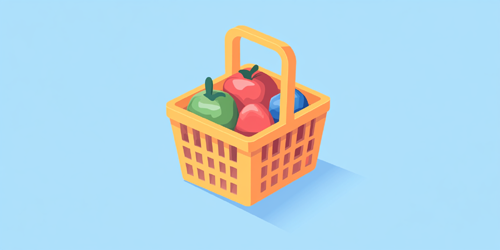
Market basket analysis is a data mining technique that identifies patterns in customer purchasing behavior by analyzing which items are frequently bought together.
Traditional Approach: Market basket analysis approaches have varied significantly over the years. Even today, many companies employ heuristic-based approaches to determine “market baskets”. In this approach, they set arbitrary thresholds for item combinations (e.g., “if two items are bought together more than 5% of the time, they are considered associated”). Other market basket analysis approaches simply involve manually examining transactional data for obvious patterns, such as which items were frequently bought together. This is a labor-intensive process, often relying on visual inspection or simple frequency counts to identify popular item pairs or combinations (like observing that milk and bread are often bought together).
Advanced Analytics Enhanced Approach: Since the late 1990’s, market basket association rules (e.g., “If a customer buys X, they’re likely to buy Y”) have often been identified using statistical methods like Apriori calculations. While more of a data-mining approach, we still categorize it under the much larger Advanced Analytics taxonomy umbrella. More recently, other more efficient AI-powered market basket analysis techniques have become available. ML algorithms like FP-Growth and AI-driven Deep Learning approaches can efficiently uncover complex relationships among products in larger datasets, and identify latent patterns across product categories that are invisible to traditional methods. Because of its efficiency and effectiveness, FP-Growth in particular is also commonly used in product recommendation systems; especially when dealing with large data sets.
Benefits: Advanced analytics transforms market basket analyses into higher-impact data driven tools, enabling marketers to design more effective cross-selling and up-selling strategies while enhancing the customer shopping experience.
- Customer Segmentation Analysis
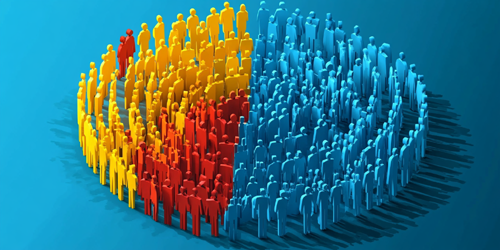
Customer Segmentation Analysis is the process of dividing a customer base into distinct groups based on shared characteristics, such as demographics, behaviors, or purchasing patterns, to tailor marketing strategies effectively
Traditional Approach: Traditional customer segmentation logic often relied on qualitative business-defined heuristics as a means for defining which customers belonged to which sub-population groups. With these often meaningless definitions in-hand, marketers would then attempt to aggregate data accordingly, often deriving incorrect insights in the process; thus delivering marketing campaigns that invariably underperformed.
Advanced Analytics Enhanced Approach: Advanced analytics based segmentation uses clustering algorithms and unsupervised learning to create more granular and dynamic data-driven and data-derived segments. For instance, various advanced analytics techniques can incorporate behavioral data, psychographics, purchase history, and hundreds or thousands of other variables to identify relevant clusters , segments, micro-segments with shared characteristics. These segments, which are amalgamations of the insights each of the variables included in their construction delivers, are a more accurate and true reflection of the dynamic nature of the segments in a given customer population.
Benefits: Advanced analytics empowers marketers to target segments with hyper-personalized campaigns, improving engagement and conversion rates. Real-time segmentation also allows brands to adapt strategies as customer behaviors shift.
- Predictive Analytics for Response Rates
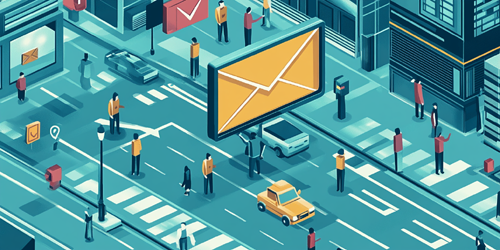
Predictive Analytics for Response Rates uses historical data and machine learning models to forecast the likelihood of a customer responding to a marketing campaign.
Traditional Approach: Traditional response rate analyses are focused on historical campaign performance, often using historical response rate averages as a basis for determining the expected response rate for future campaigns. While expedient, this approach doesn’t provide marketers with the insights needed to understand how different marketing messages, sent to different customer groups, can derive different response rates. Understanding the nature of these relationships is foundational to effective modern marketing campaign design and execution.
Advanced Analytics Enhanced Approach: Advanced analytics takes response rate analysis further with predictive models like gradient boosting and neural networks. These models analyze large quantities of historical data alongside real-time inputs to predict not only who is likely to respond but also when and how they will respond.
Benefits: Predictive analytics powered by AI and Machine Learning (ML) enables marketers to optimize campaign timing, content, and delivery channels for maximum ROI. It also reduces wasted marketing spend, by focusing available marketing dollars on higher-value prospects.
- Customer Lifetime Value (CLV) Analysis

Customer Lifetime Value (CLV) Analysis estimates the total revenue a business can expect from a customer throughout their relationship.
Traditional Approach: Calculating CLV traditionally relied on simple formulas involving average purchase value, frequency, and customer retention rates. Again, while this approach is expedient, it misses the deeper value focused nuances intrinsic to any given customer population.
Advanced Analytics Enhanced Approach: Advanced analytics based models integrate multiple data points, such as transaction history, engagement metrics, and external market conditions, as well as other model derived outputs, to forecast CLV accurately and dynamically. Reinforcement learning can even simulate the long-term impact of different marketing interventions on CLV.
Benefits: Advanced analytics driven CLV analysis helps marketers identify high-value customers early, prioritize retention efforts, and allocate resources more effectively.
- Churn Prediction
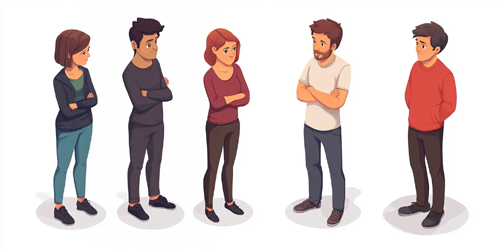
Churn Prediction identifies customers at risk of ending their relationship with a business by analyzing behavioral patterns, engagement levels, and transaction history.
Traditional Approach: Marketers traditionally identified churn risks using threshold-based manual analyses of historical purchasing trend patterns. For example, business rule driven consideration of factors like inactivity or customer complaints have often been used as early flags of potential churn.
Advanced Analytics Enhanced Approach: Advanced analytics models, such as random forests and deep neural networks, can analyze complex, non-linear relationships among variables to predict churn with greater accuracy. Natural language processing (NLP) can also analyze customer feedback to identify dissatisfaction signals.
Benefits: By accurately predicting churn, advanced analytics enables proactive retention strategies, such as personalized offers or interventions, to retain at-risk customers.
- Sentiment Analysis
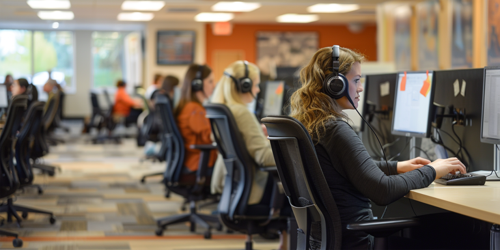
Sentiment Analysis uses natural language processing (NLP) and machine learning to evaluate customer opinions, emotions, and attitudes from text, speech, or social media data.
Traditional Approach: Sentiment analysis historically involved manual review or basic keyword analysis of customer feedback, reviews, or social media posts. This traditional approach is time consuming, expensive, error prone, and not scalable to any large degree.
Advanced Analytics Enhanced Approach: With advanced analytics, sentiment analysis leverages NLP and deep learning to interpret text, audio, and even visual data at scale. Advanced analytics based AI can detect nuances like sarcasm or mixed sentiments, providing a more comprehensive understanding of customer attitudes.
Benefits: Advanced analytics powered sentiment analysis helps brands stay ahead of reputation risks, understand customer sentiment in real time, and adjust messaging to align with public perception. More details on VentureArmor’s work in the Sentiment Analysis space can be found in this case study.
- Marketing Mix Modeling (MMM)
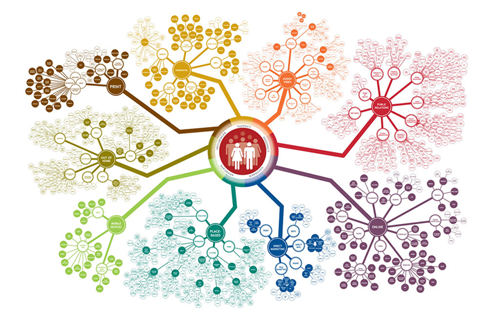
Marketing Mix Modeling (MMM) analyzes the impact of various marketing channels, such as digital ads, TV, and promotions, on sales and business performance.
Traditional Approach: Traditional MMM relied on reporting related to historical response rates for different customer segments, across different market channels, to determine the optimal marketing mix. Given that many of these customer segment definitions are qualitatively derived and sub-optimal, and that the connections between these segments and marketing performance is also qualitatively characterized… optimizing marketing mix has traditionally been very difficult.
Advanced Analytics Enhanced Approach: Advanced analytics enabled MMM uses ML to process more granular data and account for non-linear interactions among variables. These models continuously learn and adapt, providing more accurate and actionable insights, across customers, customer segments, products, prices, and promotions.
Benefits: Advanced analytics powered MMM allows marketers to dynamically determine the optimal selection of marketing channel and marketing messaging for each customer, across channels dynamically, ensuring the best possible return on investment.
- Dynamic Pricing Analysis
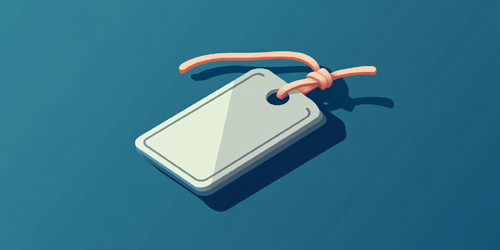
Dynamic Pricing Analysis involves adjusting product or service prices in real time based on factors like demand, competition, and customer behavior. Please see our deeper-dive discussion on Pricing here.
Traditional Approach: Traditional approaches to pricing often involve manual examination of historical data, and a heuristic driven approach to price setting, based largely on Descriptive analytics (see Analytics Maturity Curve mentioned above).
Advanced Analytics Enhanced Approach: Advanced analytics algorithms analyze real-time market conditions, competitor actions, customer behaviors, and potentially hundreds or thousands of other factors to determine optimal pricing strategies. Reinforcement learning can also simulate customer reactions to pricing changes.
Benefits: Advanced analytics driven pricing ensures competitiveness, maximizes revenue, and improves customer satisfaction by offering fair and timely pricing.
Bringing it all together: A Sum Greater Than Its Parts
By integrating the outputs of various Advanced analytics driven models—such as customer segmentation, churn prediction, and sales forecasting—marketers can unlock synergies that deliver results far beyond the individual contributions of each model.
By integrating the outputs of various advanced analytics driven models—such as customer segmentation, churn prediction, and sales forecasting—marketers can unlock synergies that deliver results far beyond the individual contributions of each model.
For instance, combining customer segmentation with churn prediction can identify high-risk segments and personalize retention efforts more effectively. Similarly, predictive sales forecasting can inform dynamic pricing models, ensuring that real-time price adjustments align with demand fluctuations and customer behavior.
The true power in an Advanced Analytics / AI-driven approach lies in the ability to merge these insights into a cohesive strategy, where the models work together to drive a more personalized, efficient, and optimized marketing operation. In doing so, AI doesn’t just enhance individual processes but supercharges entire marketing ecosystems, generating value that amplifies each component’s impact.
The Incremental Benefits of Advanced Analytics
Advanced Analytics provides several benefits over traditional approaches:
Scalability: Advanced analytics based AI and Machine Learning (ML) tools can analyze massive datasets in seconds, providing insights that were previously unattainable.
Adaptability: Machine learning models evolve with new data, ensuring relevance in dynamic markets.
Personalization: Use of Advanced Analytics techniques enables hyper-personalized marketing, improving customer engagement and loyalty.
Efficiency: Automating repetitive tasks allows marketers to focus on strategy and creativity.
In a world where data is a competitive advantage, Advanced Analytics / AI / ML driven marketing analytics offers organizations the tools they need to stay ahead. By embracing these advanced techniques, businesses can unlock new opportunities, deliver exceptional customer experiences, and achieve sustainable growth.
The evolution of marketing analytics is not just about adopting new tools—it’s about transforming how we think about data, customers, and the future of marketing.
About VentureArmor
At VentureArmor, we specialize in helping businesses unlock the power of AI to drive operational excellence and customer satisfaction. Our expertise in AI analytics and data-driven solutions enables us to deliver tailored solutions that meet the unique needs of our clients. Contact us to learn more about how we can help your organization achieve its goals through the strategic application of AI. VentureArmor: Delivering ROI with AI.