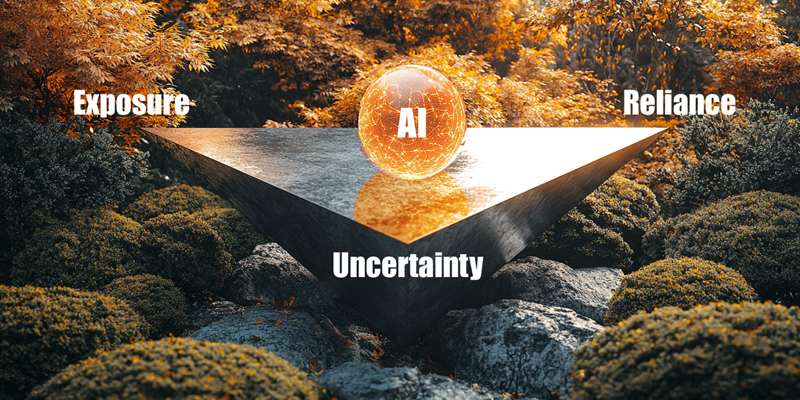
In an era where artificial intelligence (AI) and machine learning (ML) are transforming industries, ensuring robust governance of these models is crucial. Model governance has long been a well-established practice in the financial services industry, where model risk management is guided by three core risk concepts: Exposure, Reliance, and Uncertainty. These principles provide a strong foundation for governing both classical ML models and the more recent advancements in Generative AI (GenAI).
By applying these well-defined risk concepts, organizations can develop structured governance frameworks that mitigate risks and ensure AI-driven decision-making remains ethical, reliable, and transparent. In this article, we will explore these three categories of model risk and discuss best practices for mitigating them in both classical ML and Generative AI models.
Understanding Model Risk: Exposure, Reliance, and Uncertainty
Financial institutions have long considered model risk within three broad categories: Exposure, Reliance, and Uncertainty. While these concepts have been applied to statistical and ML models for decades, they can and frequently are applied to assess and govern Generative AI models.
Exposure Risk: The Cost of Getting It Wrong
Exposure risk refers to the financial and reputational risks an organization assumes when implementing a model. This risk is particularly high when a model is directly tied to critical financial decisions, such as loan underwriting, fraud detection, or trading strategies.
For example, a machine learning model designed to assess loan risk carries significant exposure risk. If the model is flawed—due to issues in data quality, bias, or inadequate validation—the financial consequences could be severe for the company that implements this model. In particular, improperly underwriting loans may not only lead to substantial direct financial losses from making bad loans based on the model’s bad recommendations, additional and often very substantial regulatory fines can also be levied against the organization if the model facilitates adverse impact or illegal recommendations (e.g. discriminatory lending practices). Aside from these very real and immediate financial consequences, poor quality models can drive decisions that also damage a company’s brand and reputation within the market, further eroding profitability and revenue generating potential in the future.
When assessing exposure risk, organizations should:
- Conduct rigorous testing and validation: Models should be rigorously stress-tested to identify weaknesses before deployment. The rigor of this testing should be comparable to the risk that the roll-out of a model presents to the organization.
- Implement fairness and bias assessments: AI models, particularly in highly regulated industries, must be scrutinized for bias and ethical concerns.
- Ensure regulatory compliance: Compliance with frameworks such as SR 11-7 (for model risk management in banking) helps mitigate regulatory exposure. The US Federal Reserve’s Model Risk Management Guidance (PDF) provides an excellent overview of the topic.
These same principles apply to Generative AI. For instance, if a financial institution deploys a GenAI chatbot to provide customer investment advice, any misleading or incorrect outputs could result in regulatory penalties, lawsuits, and customer distrust.
Regardless of the model type; classical statistical model, machine learning model, deep learning model, generative AI model, the means and measures of assessing exposure risk are the same. Regardless, if a particular model application is deemed to be “high risk”, a variety of measures can be put into place to mitigate these risks. Significant foresight and planning is required, however, to ensure that these mitigation practices are effective..
Reliance Risk: The Perils of Overdependence
Reliance risk refers to how heavily or broadly an organization depends on a given model for decision-making. While similar to exposure risk, reliance risk focuses more on the degree to which an organization integrates a model into its operations, rather than the direct financial impact of errors.
Consider a predictive econometric model used for financial forecasting. While the model itself may not trigger immediate financial transactions, if its predictions inform broader business strategies, a flawed model can lead to poor investment decisions or operational inefficiencies. Similarly, reliance risk is particularly high when a model is used as an input into other critical systems.
To mitigate reliance risk, best practices include:
- Model redundancy and alternative decision pathways: Avoid sole reliance on a single model by using complementary models or expert judgment.
- Continuous performance monitoring: Deploy real-time monitoring to detect model drift and unexpected deviations.
- Periodic reassessment and recalibration: Regularly update models to reflect new data trends and avoid reliance on outdated assumptions.
Generative AI presents unique challenges in reliance risk. If an organization incorporates AI-generated content into the evaluation of legal documents, contracts, or in the execution of risk assessments for example, their reliance on potentially non-factual outputs could have serious consequences.
Organizations should employ rigorous quantitative review processes comparable to the level of their reliance risk for a given model, as well as human validation checkpoints, to ensure model outputs are trustworthy for any large-scale roll-out.
Uncertainty Risk: The Black Box Problem
Uncertainty risk pertains to the inherent transparency and interpretability of a model. For example, traditional statistical models, such as logistic regression or decision trees, provide clear, interpretable outputs. However, more complex machine learning models—especially deep learning and neural networks—often act as black boxes, making it difficult to understand how decisions are made.
This issue is even more pronounced in Generative AI models, where outputs are not deterministic and may be difficult to validate. A large language model (LLM), for instance, generates responses based on training data, but it is often challenging or impossible to trace the reasoning behind specific outputs. This opacity increases the risk of biased, misleading, or incorrect content being generated.
Best practices for managing uncertainty risk include:
- Explainability and interpretability tools: Utilize techniques such as SHAP (Shapley Additive Explanations) and LIME (Local Interpretable Model-agnostic Explanations) to provide insights into model behavior. While these techniques are well suited to evaluate the influence of structured model input variables associated with traditional statistical, machine learning, and even some deep learning analyses, they have limited or no ability to provide insights into the influence of unstructured data/variables inputted into Generative AI models.
- Robust documentation and audit trails: Maintain clear documentation of model design, training data, and key decision points to improve transparency.
- Human-in-the-loop oversight: Implement checkpoints where humans validate high-risk model outputs before they influence key decisions.
For Generative AI, uncertainty risk requires even more stringent controls. AI-generated content should always be flagged as such, and companies should implement review layers to assess factual accuracy, bias, and appropriateness.
Extending Traditional Model Governance to Generative AI
While the financial services industry has long applied these governance principles to traditional models, the rise of Generative AI requires adapting and extending these frameworks. GenAI models present innately high uncertainty risks by default, and their exposure and reliance risks vary depending on their applications.
To align with best practices, organizations should:
- Adopt structured governance frameworks: Implement and use well established and traditional model risk management principles and apply them to GenAI.
- Ensure appropriate AI model explainability: As much as the end-application needs dictate, leverage models that are sufficiently explainable. While this can be extremely challenging when dealing with GenAI models, leverage research on explainable AI (XAI) to enhance transparency in generative models. Balance the explainability of your model with the implications of your model’s Exposure and Reliance
- Implement safeguards against hallucinations: Generative models can create false or misleading information, requiring mechanisms to validate and fact-check outputs.
Conclusion
The governance of AI and machine learning models is a critical discipline, especially as organizations increasingly depend on AI-driven decision-making. By leveraging the well-established principles of Exposure, Reliance, and Uncertainty risk—developed in the financial services industry—businesses can effectively manage both classical ML models and the emerging risks posed by Generative AI.
Companies that adopt these best practices will be better positioned to navigate regulatory landscapes, mitigate financial and reputational risks, and build trust in AI-powered systems.
About VentureArmor
If your organization is looking to strengthen its AI model governance infrastructure, VentureArmor AI is here to help. We specialize in setting up governance frameworks, establishing model risk protocols, and conducting comprehensive AI audits. Our analytical leads have led similar functions at several of the largest and most heavily regulated Financial Services companies in the US. Contact us today to learn more about how we can support your AI governance needs.
At VentureArmor, we specialize in helping businesses unlock the power of AI to drive operational excellence and customer satisfaction. Our expertise in AI analytics and data-driven solutions enables us to deliver tailored solutions that meet the unique needs of our clients. Contact us to learn more about how we can help your organization achieve its goals through the strategic application of AI. VentureArmor: Delivering ROI with AI.