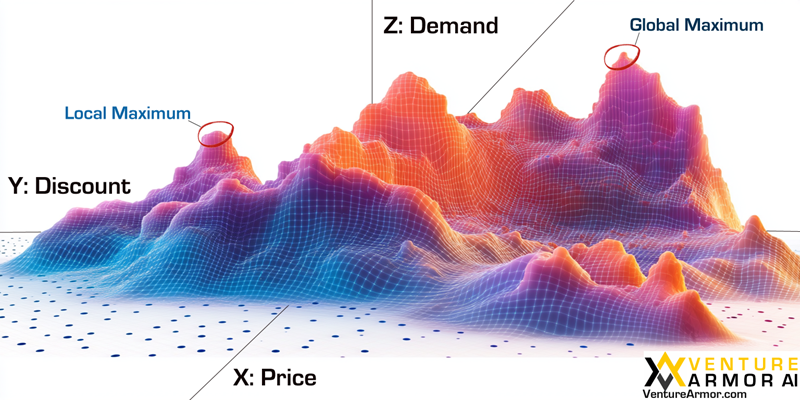
Pricing plays a crucial role in the success of any business, whether in retail or wholesale. The strategies behind retail and wholesale pricing vary, as they cater to different segments of the supply chain. Wholesale pricing is focused on selling goods in large quantities to retailers or other businesses, while retail pricing targets end consumers. Let’s explore how these pricing strategies are determined and the factors influencing them.
Background: Understanding Retail and Wholesale Pricing
Wholesale Pricing
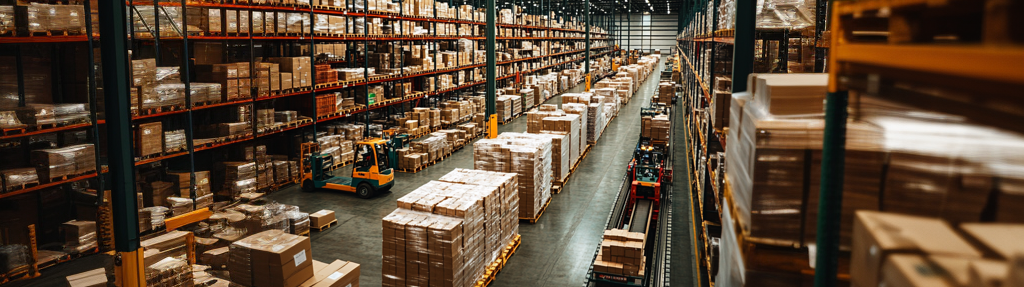
Definition:
Wholesale pricing is the price set by manufacturers or distributors when selling products in bulk to retailers, resellers, or other businesses.
Key Elements of Wholesale Pricing:
- Cost of Goods Sold (COGS):
The starting point for any wholesale price is the COGS, which includes the cost of raw materials, manufacturing, labor, and overhead expenses. A wholesaler must ensure the wholesale price covers these costs to maintain profitability. - Profit Margins:
Wholesalers add a profit margin to the COGS to determine the final price. Typical wholesale profit margins range from 15% to 50%, depending on the industry, product, and competition. - Volume Discounts:
Wholesalers often offer tiered pricing based on order size. Larger orders receive steeper discounts, incentivizing bulk purchases and fostering long-term business relationships. - Market Trends and Competition:
Wholesale pricing must be competitive. Businesses frequently analyze industry trends, competitor pricing, and customer demand to adjust their prices while maintaining profitability. - Logistics and Distribution Costs:
Shipping, storage, and handling fees are incorporated into the wholesale price. Businesses may either pass these costs to the retailer or absorb them, depending on their strategy.
Traditional Heuristic Methods for Setting Wholesale Prices:
- Keystone Pricing:
A common wholesale pricing strategy where the cost is doubled (COGS × 2) to establish the wholesale price. This method works well in industries with stable costs and predictable demand. This pricing approach traces back to the jewelry trade in the late 19th century. A publication named ”The Keystone” introduced the concept to jewelers - Cost-Plus Pricing:
Adding a fixed percentage or dollar amount to the COGS ensures profit margins are met. - Competitive Pricing:
Businesses analyze competitor pricing and set their wholesale prices slightly below or within the same range to attract buyers.
Retail Pricing

Definition:
Retail pricing is the price charged to consumers for a product or service. This price is typically higher than the wholesale price to account for operating costs and other expenses.
Key Elements of Retail Pricing:
- Wholesale Cost:
Retail pricing begins with the wholesale cost—the price paid by the retailer to acquire the product. - Operating Expenses:
Retailers factor in expenses such as rent, utilities, wages, marketing, and inventory management. These costs vary widely depending on the business size and location. - Markup:
The markup is the amount added to the wholesale cost to cover operating expenses and ensure profitability. Markups can vary significantly, with some industries operating on slim margins (e.g., grocery stores) and others with higher margins (e.g. luxury goods). - Consumer Demand:
Retail pricing must reflect the perceived value of the product. High demand or unique products may justify higher prices, while excess inventory or competitive markets may necessitate price reductions. - Psychological Pricing:
Strategies like setting prices at $19.99 instead of $20.00 can influence consumer behavior. This approach leverages consumer psychology to make products appear more affordable.
Traditional Heuristic Methods for Setting Retail Prices:
- Keystone Markup:
Retailers double the wholesale price (Wholesale Price × 2) to determine the retail price. - Manufacturer’s Suggested Retail Price (MSRP):
Some manufacturers provide a recommended retail price, ensuring consistency across retailers. This approach is common in electronics, automotive, and branded goods. - Discount Pricing:
Temporary reductions, sales, or promotions attract customers and increase volume sales. Retailers must balance discounts with profit margins to avoid losses.
Comparing Wholesale and Retail Pricing
Wholesale Pricing | Retail Pricing | |
Target Audience | Businesses, resellers, retailers | End consumers |
Volume | High-volume sales | Low-volume, individual sales |
Profit Margins | Lower margins | Higher margins |
Complexity | Focused on production and distribution costs | Accounts for consumer behavior and marketing |
Adjustability | Based on contracts and bulk orders | Flexible for promotions, demand, and seasons |
Challenges in Pricing
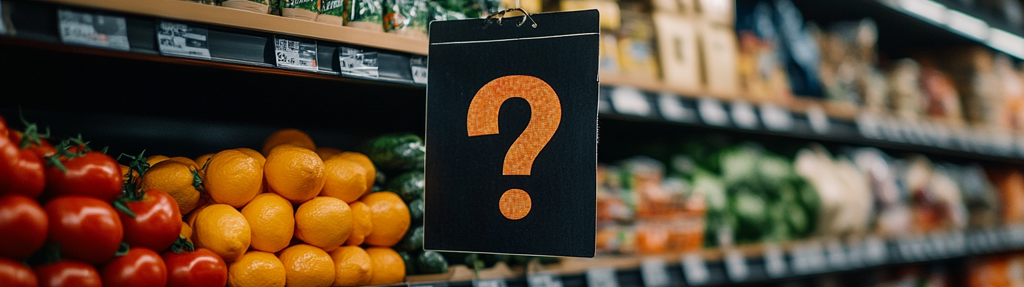
When wholesalers and retailers set prices, they need to keep a myriad of simultaneous considerations in mind, as they attempt to achieve their desired outcomes (e.g. profit maximization) while still operating within certain constraints (e.g. in-stock levels, sales volumes, and more). Some of these considerations include:
- Balancing Profitability and Competitiveness: Both wholesalers and retailers must strike a balance between offering competitive prices and maintaining healthy profit margins.
- Economic Fluctuations: Inflation, currency fluctuations, and changes in raw material costs impact pricing strategies.
- Market Saturation: High competition may force businesses to lower prices, affecting profitability.
- Evolving Consumer Preferences: Retailers, in particular, must adapt to changing trends, preferences, and purchasing power.
- Ever Changing Competitive Pressures: The constantly changing competitive environment that both wholesalers and retailers operate in can rapidly shift customer demand. This is true in terms of competitive price changes, competitor discounts and promotions, and competitor geographic positioning (Store and DC locations).
- Supply Chain: The changing availability of products within the supply chain at various times can affect both wholesaler and retailer ability to stock and sell products.
Setting the right price for a product, with the right discount type (if any), at the right discount amount (if any), given the myriad of the above considerations for any given product, being sold at any given location can be EXTREMELY complicated. Hundreds or thousands of variables need to be simultaneously considered, to make an optimized decision. Finding this massively multi-dimensional balance via manual means is physically impossible.
Setting the right price for a product, with the right discount type (if any), at the right discount amount (if any), given the myriad of the above considerations for any given product, being sold at any given location can be EXTREMELY complicated. Hundreds or thousands of variables need to be simultaneously considered, to make an optimized decision. Finding this massively multi-dimensional balance via manual means is physically impossible. This is why companies have turned to AI and large-scale compute to help solve these otherwise intractable problems.
Modern Pricing: Tackling Problem Complexity with AI
AI-based analytics can provide businesses with actionable insights to address pricing challenges effectively, enabling data-driven pricing strategies that maximize decision making at the multi-dimensional intersections between profitability, customer satisfaction, and hundreds/thousands of other considerations.
Problem Complexity
To illustrate the complexity of the problem space, consider the following. If one wanted to understand demand dynamics for a product by considering just one variable, the price charged for that product and the resulting demand, producing an X-Y scatter plot of this relationship might look something like this:
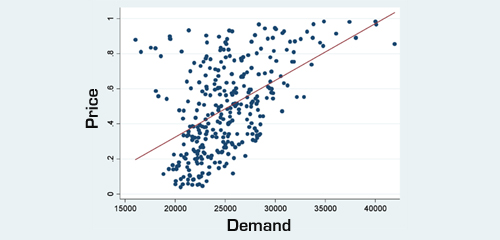
While this is perhaps a good starting point for analysis, given the relatively diffused spread of the dots in this graph, there appears to be a lot going on that is not explained by just considering price alone. What if we were to include another variable, discount amount? If we did, then the graph might look something like this:
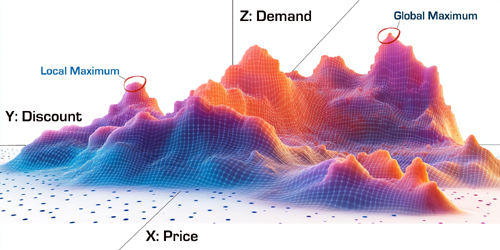
This appears to be an improvement, and we can use this visual to get a better impression of the complexity of the space. In this visual, we can see certain combinations of price and discounts that yield localized demand benefit (Local Maximums), and other points that globally seem to be the best combination of price and discount (Global Maximums). It is important to note that while the above illustration might represent a true visualization of the historical relationship between these three variables, the applicability of this characterization to future demand forecasts would be probabilistic. Said differently, imagine a fuzzy/blurry version of the above surface, where the projected demand at any given discount and price combination would be found in that haze.
Ultimately, pricing decision makers want to reduce the amount of fuzziness in this probability surface. To accomplish this, decision makers often introduce additional variables/dimensions into the analytical process – sometimes hundreds or thousands of additional variables – in an attempt to capture the influences of those variables on demand dynamics. Doing this produces a complex massively multidimensional surface, with hundreds or thousands of dimensions, that is impossible to visualize, and extremely difficult to explore, to find the optimal pricing/other points within.

Despite the challenges involved in exploring this massively multidimensional space, there are two ways that businesses analytically approach this complexity: 1) Price Elasticity based analytics and 2) Dynamic/Real-Time Pricing based analytics.
Option 1: Price Elasticity & Outcome Simulation
By analyzing historical sales data, businesses can build AI models to understand how changes in price, discount type, discount amount, promotion, shelf placement, and more can affect demand for their products, in the context of the myriad of other operational considerations that can impact demand.

Once these AI models are built, other AI tools can be employed to explore the dynamics of the relationships between the variables represented within the model. You can think of it like a switchboard with dozens/hundreds/thousands of dials; each dial representing a different variable or decision factor for a business, and the settings for this dial being the different values that could be set for this variable. The machine behind the switchboard is the model. The dials are the controls for the model (i.e. the various price, discount, and other decision factors a business can make). Finding the optimal setting for each dial, such that an optimized outcome is produced, can be extremely complex. However, AI can be used to intelligently and efficiently explore the various possibilities very quickly, and achieve optimized “dial” recommendations.
Again, taking this approach entails implementing 1) An AI-based representation of the relationship between demand and other factors for a given product or set of products, and 2) The technical ability to intelligently explore the dynamics of this model, to find the optimal decision points. Both of these builds can be complex. While this approach can produce very robust and valuable insights, building and maintaining AI models that are accurate representations of business dynamics can be a significant undertaking. Because of these challenges, some businesses choose to take a different approach: Dynamic/Real-Time Pricing.
Option 2: Dynamic / Real-Time Pricing
Dynamic pricing optimization can be implemented via a number of often very expensive proprietary applications. These systems use decision-making algorithms to determine the most appropriate price for a product or service in real time, based on dynamic A/B testing.
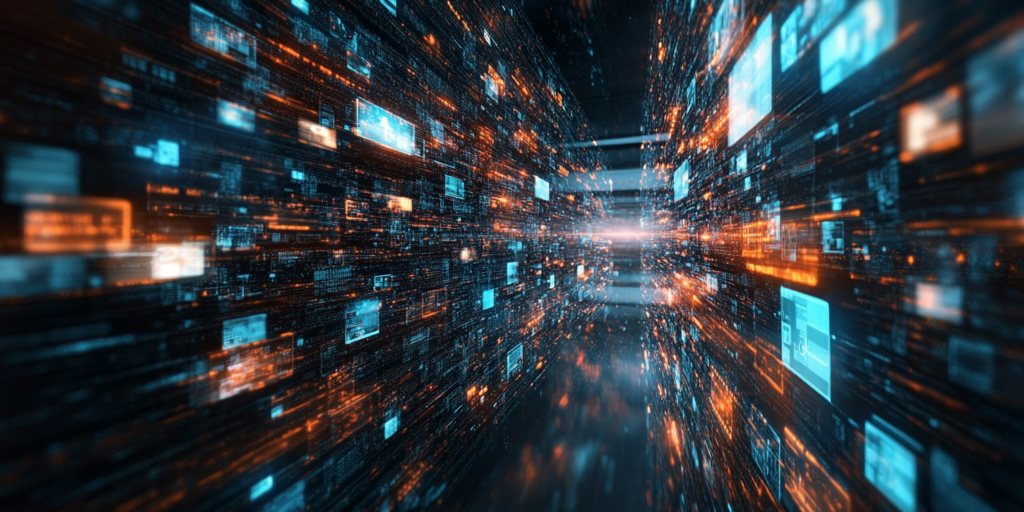
While no master AI model is often produced by these systems, the incremental probability of certain customers acquiring certain products is computed (ex: Naïve Bayes), and is used to incrementally optimize future pricing and offer presentment decisions. Here’s how it works:
- A/B Testing and Experimentation
- Optimization within these systems are based on large scale dynamically designed and executed A/B testing experiments, each reflecting different pricing and offer presentment strategies across different scenarios, to achieve optimal outcomes. Businesses can test varying price points, discounts, or bundles to identify the most effective approach for driving conversions and maximizing revenue across different situations.
- Real-Time Decisioning
- These systems leverage real-time decision-making to dynamically adjust pricing for individual customers. They consider data such as current demand, customer interactions, and external market conditions to suggest the best price at any given moment.
- For instance, if a customer shows interest in a product but hesitates to purchase, the system might offer a discount or promotional price to nudge them toward conversion.
- Personalization at Scale
- Using its customer profile and interaction history, these systems personalize pricing strategies for individual customers. Factors like purchase history, loyalty status, and willingness to pay (inferred from past behavior) influence the pricing recommendations.
- Example: A loyal customer might receive a lower price or bundled offers, whereas a new customer may be shown a standard or premium price.
These types of systems are often used to manage product and offer presentment on websites, where real-time feedback can be readily obtained. They can also be used to manage offline brick-and-mortar aspects of sales as well.
Summary
Advanced wholesale and retail pricing involves navigating the complexities of setting optimal prices for businesses and consumers. While wholesale pricing prioritizes bulk sales with considerations like COGS, profit margins, and volume discounts, retail pricing focuses on consumer demand, psychological pricing, and operational expenses. Traditional heuristic methods, such as keystone or cost-plus pricing, have been effective, but modern AI-driven approaches are increasingly driving pricing decisions in business.
Competing effectively against these automated and AI driven systems by manual means is simply not possible. They will beat human-based decisions 999 times out of 1000.
Competing effectively against these automated and AI driven systems by manual means is simply not possible. They will beat human heuristic-based decisions 999 times out of 1000. The human minds is not able to factor-in the hundreds or thousands of considerations needed to optimize outcomes, while simultaneously balancing other operational constraints. There are simply too many “dials and levers” to consider. While this kind of complexity is still a challenge even for computers, it is a challenge that they are particularly adept at tackling.
By leveraging techniques like price elasticity modeling or dynamic/real-time pricing, however, businesses can optimize outcomes across a vast array of variables. These advanced methodologies enable smarter, data-driven decisions, maximizing profitability while meeting market and business demands.
About VentureArmor
At VentureArmor, we specialize in helping businesses unlock the power of AI to drive operational excellence and customer satisfaction. Our expertise in AI analytics, pricing, and data-driven solutions enables us to deliver tailored solutions that meet the unique needs of our clients. Contact us to learn more about how we can help your organization achieve its goals through the strategic application of AI. VentureArmor: Delivering ROI with AI.