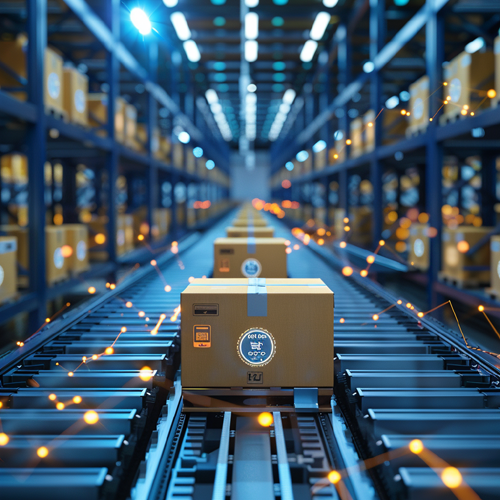
Introduction
Shrink—inventory loss due to theft, error, or damage—is a perennial challenge for businesses operating large-scale distribution centers (DCs). For a Fortune 500 client with a nationwide network of DCs, shrink had been a significant operational issue, costing over $100 million annually. The traditional approach of manually identifying and addressing shrink was reactive, labor-intensive, and insufficient for preventing future losses. Our data scientists partnered with this client to develop and implement an AI-powered shrink mitigation solution, transforming their operations and saving millions of dollars.
The Challenge
The client faced persistent and costly issues related to shrink across their distribution centers. Key challenges included:
- High Shrink Costs: Shrink expenses exceeded $100 million annually, significantly impacting profitability.
- Labor-Intensive Processes: Teams spent 50% of their time analyzing inventory reports to identify shrink and the other 50% remediating identified issues, leaving little room for proactive measures.
- Reactive Approach: The company addressed shrink only after it occurred. Efforts to remediate one issue would lead to new problems emerging elsewhere, creating a “whack-a-mole” effect.
- Lack of Root Cause Understanding: The absence of a comprehensive analysis of shrink’s root causes hindered their ability to implement preventative strategies.
To achieve meaningful and lasting results, the company needed a fundamental shift from reactive remediation to proactive prevention.
The Solution
VentureArmor Data Scientists designed and implemented an advanced AI/ML solution tailored to address the client’s shrink challenges comprehensively. The solution featured three core components:
- Defining the Target: We began by collaborating with the client to define shrink with precision. This step ensured alignment on the scope of the problem and enabled us to set measurable objectives.
- Data Aggregation: We aggregated and harmonized data from multiple sources, encompassing hundreds of simultaneous variables, including inventory levels, operational metrics, and environmental factors, for each DC.
- AI-Powered Analysis: Using machine learning frameworks, we analyzed these variables (600+ simultaneous operational variables) to identify connections, patterns, and root causes of shrink. The models processed data monthly and delivered actionable insights to the client.
This AI/ML-driven approach shifted the client’s focus from merely detecting shrink to understanding and addressing its underlying causes.
Implementation
The implementation phase was characterized by meticulous planning, collaboration, and technical execution. Key steps included:
- Requirement Definition: We partnered with stakeholders across the client’s organization to define the scope of the project, ensuring that all relevant factors were considered and aligning on what constituted shrink.
- Data Integration and Preparation: Data from multiple systems were aggregated and pre-processed for analysis. This required extensive data cleansing and validation to ensure the quality and consistency of the inputs.
- Model Development and Deployment: We developed machine learning models capable of uncovering nuanced relationships between variables and shrink. These models were deployed to provide monthly insights into high-risk areas and their underlying causes.
- Reporting and Visualization: Insights were delivered through intuitive dashboards, enabling teams to identify root causes and prioritize remediation efforts.
- Change Management: We worked closely with the client to reorganize their teams, ensuring that insights from the models were actionable and seamlessly integrated into their operations.
Results
The AI/ML-driven solution produced transformative results for the client, addressing shrink in a way that was both effective and sustainable:
- Significant Cost Savings: Shrink was reduced from the organization’s top operational challenge to a minor concern, saving millions of dollars annually.
- Operational Efficiency: Teams that previously spent half their time identifying shrink and half their time remediating it were restructured. With insights from the AI models, these teams could dedicate 100% of their time to targeted remediation, maximizing their impact.
- Proactive Risk Management: The models provided monthly insights into shrink risks, allowing the client to address issues before they escalated.
- Root Cause Resolution: By focusing on the root causes of shrink, the client implemented lasting solutions that prevented future losses.
- Scalability: The framework was designed to adapt to new data and evolving challenges, ensuring its long-term utility.
The implementation of this AI/ML solution fundamentally changed the organization’s approach to shrink, delivering a powerful return on investment.
Conclusion
This case study underscores the transformative potential of AI/ML in addressing complex operational challenges like shrink. By shifting from a reactive approach to one focused on proactive prevention, our client not only achieved substantial cost savings but also enhanced their operational efficiency and organizational resilience.
For businesses struggling with shrink or similar challenges, this example illustrates the value of leveraging advanced analytics to uncover root causes and drive meaningful change. VentureArmor specializes in delivering tailored solutions that align with your unique needs, ensuring measurable and sustainable results. Let’s work together to revolutionize how your organization approaches its biggest challenges.
About VentureArmor
At VentureArmor, we specialize in helping businesses unlock the power of AI to drive operational excellence and customer satisfaction. Our expertise in AI analytics and data-driven solutions enables us to deliver tailored solutions that meet the unique needs of our clients. Contact us today to learn more about how we can help your organization achieve its goals through the strategic application of AI.